Enhancing Analytical Infrastructure and Reducing Fraudulent Activities
Led the shift of analytical infrastructure, enhancing data resources and decreasing update errors by 90%. Increased identifiable traffic sources from 60% to 95%. Played a crucial role in reducing fraudulent responses from 28% to 1-4% by strengthening moderation and creating a fraud analytics direction. Contributed to a project reducing the cost of applications from groups by 70% and reducing vacancies in central Moscow from 20% to 6%.
About expert
A Lead Product Analyst at vk.com.
Pavel applied their knowledge of Product analysis and Data analysis in Social Media and HRTech on the following markets: Russia.
Pavel built products like VK Job and VK Rabota using the variety of tools such as tool Superset, Redash, Tableau and Google Analytics.
Other cases by Pavel
Building an Integrated Automated Sales Analytics System
Developed an integrated automated online and offline sales analytics system, utilizing various tools like GA, ExpertSender, Dynamic Call Tracking, Naumen, and 1C. Reduced BigQuery costs by over three times and improved the effectiveness of advertising campaigns by 8-10%.

Similar cases
Setting up efficient customer support
Organized pre-launch product UX testing, regular users research and new features backlog prioritization
Organized the company's participation in high-profile contests, resulting in Nimb becoming a winner of Smart City Expo worldwide Congress’s call for solution in the “Safe Cities” category and a finalist of Xprize Women’s Safety competition
Set up efficient client support

Data Flow Enhancement and Efficient Processing through Large Language Models (LLMs)
In the corporate sector, text data flows swiftly, emanating from diverse sources in various formats, destined for multiple endpoints and actions. This continuous stream of data necessitates management through structuring, making it easy to interpret and analyse, and ultimately directing it to the appropriate user or applying the insights obtained. I utilised several large language models (LLMs) streamline and enhance this process:
A question-answering LLM, enabling users to inquire about the content of each document within the flow.
A summarization LLM, offering a concise overview of each document.
An NLP model for sentiment analysis, determining the sentiment of each document.
An NLP model that calculates an absolute similarity score among all documents.
Clustering, which groups similar documents together and simultaneously identifies outlier documents.
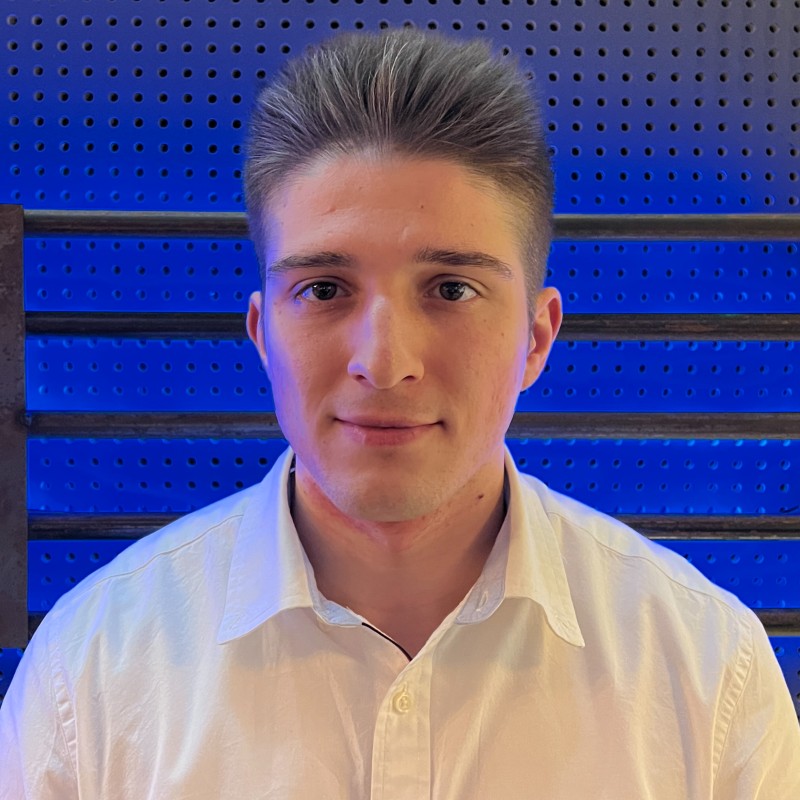